
Fast.ai
Introduction: Discover Fast.ai's free courses and open-source library for practical deep learning implementation. Learn cutting-edge techniques through hands-on projects and community-driven education.
Pricing Model: Free educational resources (Donation-supported) (Please note that the pricing model may be outdated.)
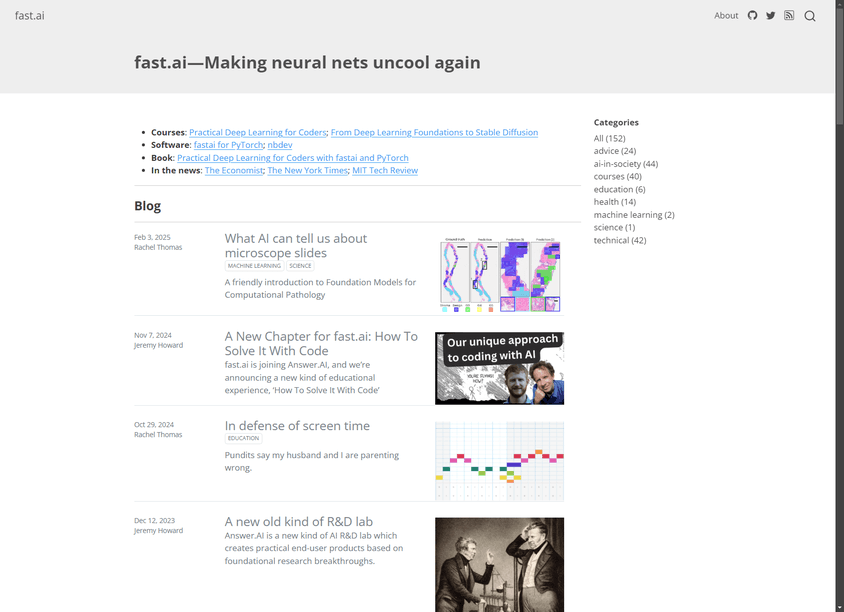
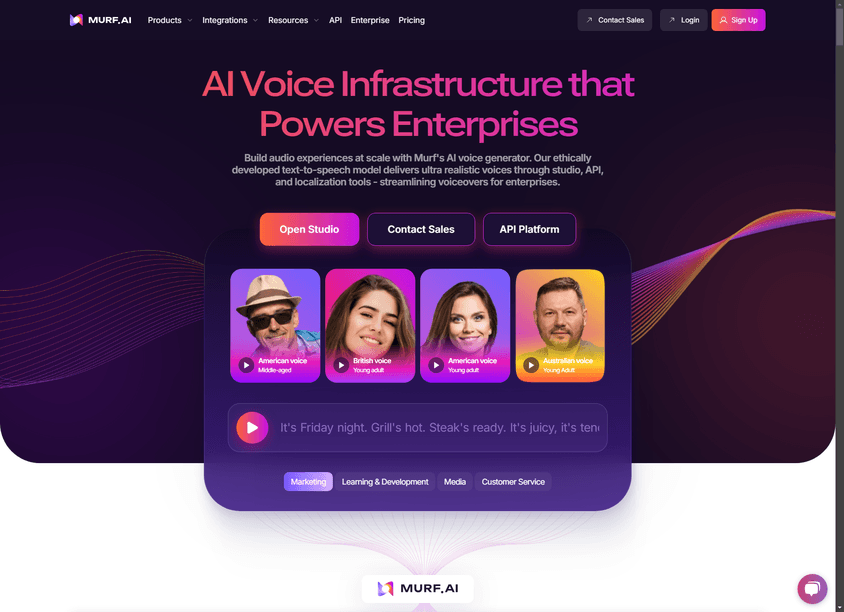

Murf AI
Murf AI is a versatile text-to-speech platform that transforms text into realistic, human-like voiceovers. With over 200 voices across 20+ languages, it offers solutions for various applications, including eLearning, marketing, and media. Key features include voice cloning, AI dubbing, and seamless integration with tools like Canva and Google Slides.
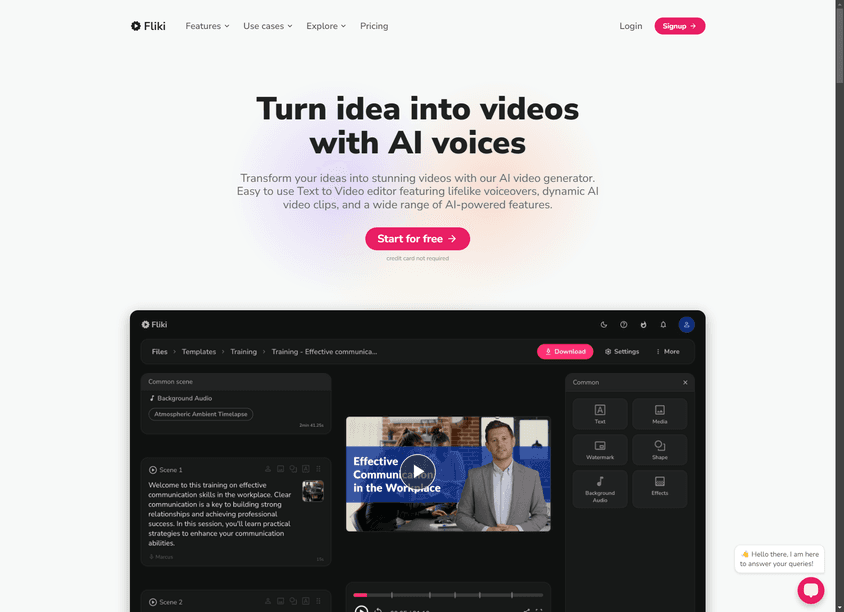

Fliki AI
Transform text into engaging videos using Fliki AI's text-to-video generator. Features 2000+ ultra-realistic voices in 80+ languages, voice cloning, and HD video creation. Ideal for content creators and marketers.
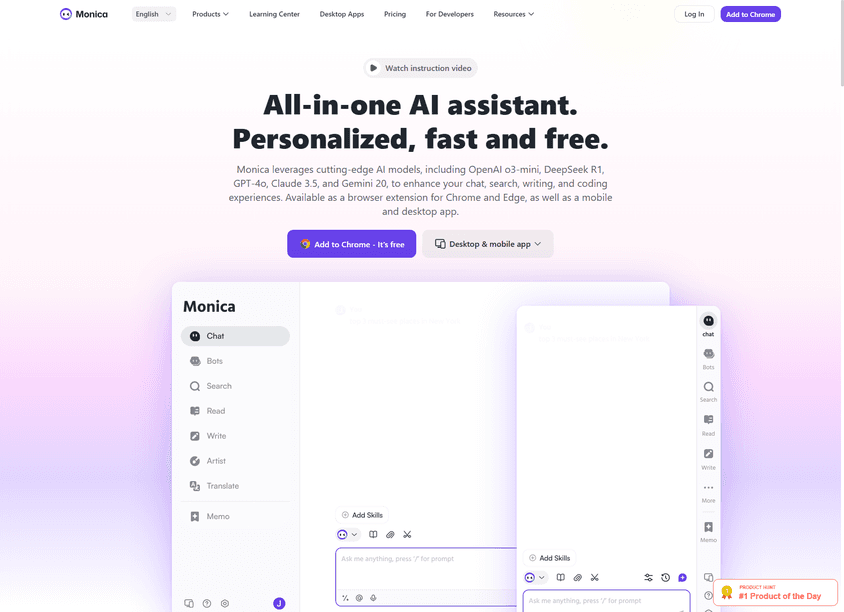

Monica
Discover Monica AI - a versatile productivity suite offering GPT-4o, Claude 3.5 Sonnet integration, SEO-optimized writing tools, real-time translation, and cross-platform support for enhanced workflow efficiency.
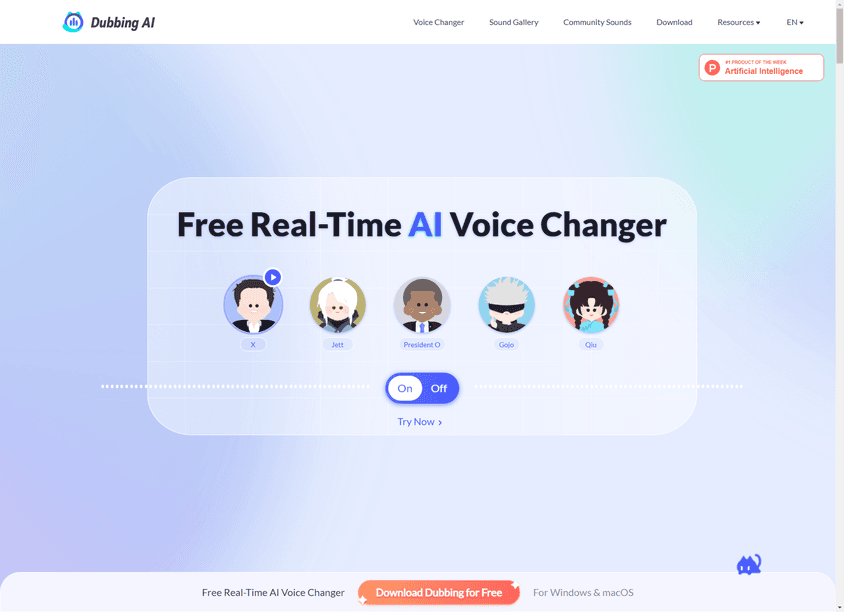

Dubbing AI
Dubbing AI offers a powerful real-time voice changer with over 1,000 unique voices, low latency, and easy-to-use features for gamers, streamers, and content creators.
In-Depth Analysis
Overview
- Deep Learning Framework: Fast AI is an open-source deep learning library built on PyTorch that provides layered APIs for both practitioners seeking state-of-the-art results and researchers requiring customizable components.
- Educational Ecosystem: Developed alongside practical courses by Jeremy Howard and Rachel Thomas, Fast AI emphasizes accessibility through its 'top-down' teaching approach paired with production-ready code implementations.
- Domain-Specific Optimization: Offers specialized toolkits for vision, text processing, tabular data analysis, and collaborative filtering with integrated best practices from recent research papers.
Use Cases
- Medical Imaging Analysis: Researchers leverage Fast AI's GPU-optimized vision modules with DICOM support for rapid prototyping of diagnostic models using limited datasets.
- Transfer Learning Deployment: Computer vision teams achieve production-grade image classification models in under five code lines using pretrained architectures like ResNet variants.
- NLP Pipeline Development: Natural language processing engineers utilize ULMFiT-inspired workflows from Fast AI's text module for domain-specific language model fine-tuning.
- Recommendation Systems: E-commerce platforms implement collaborative filtering APIs to build personalized product suggestion engines with implicit feedback handling.
Key Features
- Intelligent Defaults System: Automatically selects optimal loss functions, validation strategies, and transfer learning parameters based on data characteristics through unified classes like Learner and DataLoaders.
- Modular Architecture: Combines PyTorch flexibility with high-level abstractions through decoupled components including XResNet blocks, merge layers for custom connections, and GPU-optimized vision operations.
- Advanced Training Utilities: Implements progressive resizing for accelerated image processing, discriminative learning rates for fine-tuning efficiency, and novel callback systems enabling mid-training parameter adjustments.
Final Recommendation
- Ideal for Applied ML Teams: Combines rapid prototyping capabilities with research-grade customization options suitable for startups to enterprise deployments.
- Recommended for Educational Programs: Integrated course materials paired with battle-tested code patterns make it exceptional for teaching practical deep learning concepts.
- Essential for PyTorch Practitioners: Serves as complementary toolkit enhancing native PyTorch workflows through optimized training loops and architectural best practices.