What makes deepseek's ai models more cost-effective than competitors
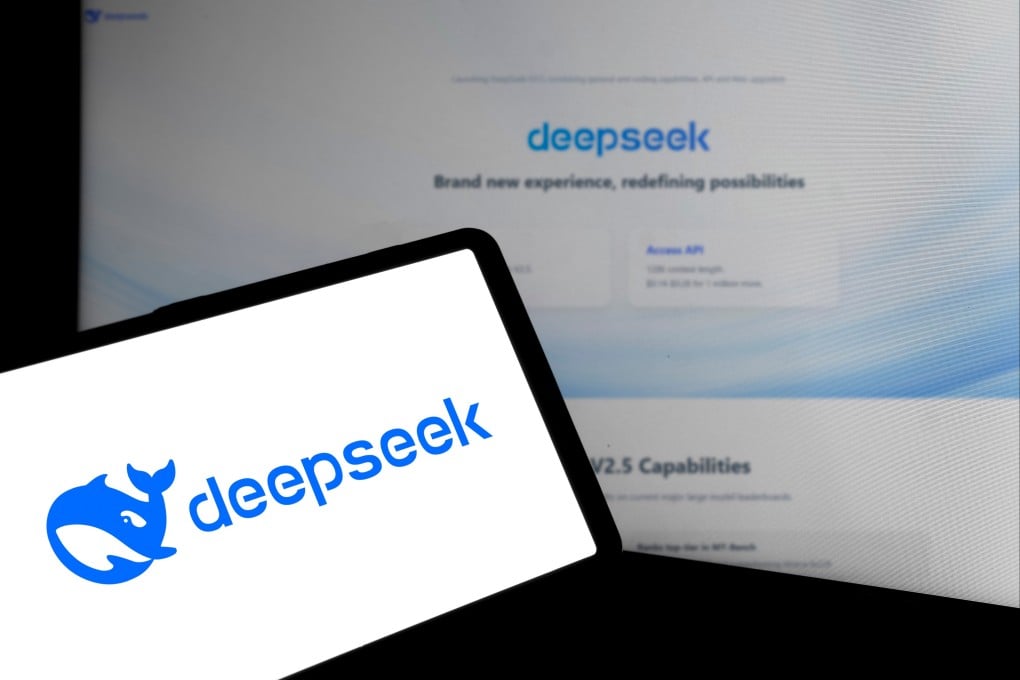
DeepSeek, a Chinese AI startup founded in 2023 and operating under the quantitative hedge fund High-Flyer, has made a significant impact on the AI landscape. In just 14 days, DeepSeek reached 1 million users, and within 20 days, it amassed 10 million users, highlighting the rapid adoption of its models. This report delves into the factors that contribute to DeepSeek's cost-effectiveness, examining its model architecture, training data, pricing strategies, and deployment methods.
DeepSeek's Innovative Architecture
DeepSeek's AI models achieve comparable performance to its competitors while requiring potentially lower hardware specifications. This is achieved through a foundation of efficiency. The company utilizes a Mixture-of-Experts (MoE) architecture, which selectively activates different parts of the model depending on the task at hand. This approach significantly reduces the computational load compared to traditional dense models, where all parameters are active for every input. DeepSeek also incorporates Multi-Head Latent Attention (MLA), an optimized attention mechanism that enhances performance while minimizing resource consumption
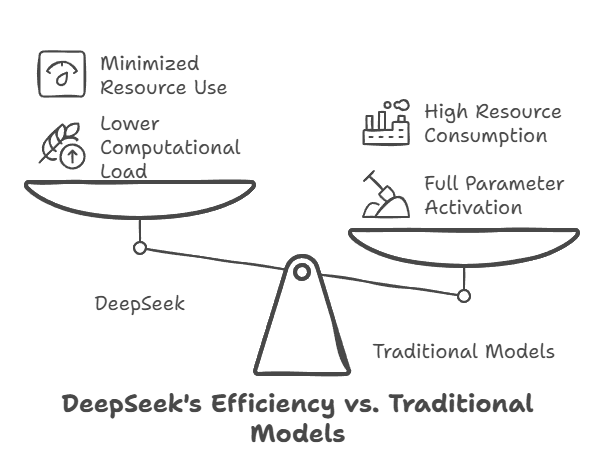
Efficient Training Data and Methods
DeepSeek's approach to training data and methods further contributes to its cost-effectiveness. The company leverages reinforcement learning (RL) and supervised fine-tuning (SFT) to achieve high performance with less data and computational resources. This approach allows the model to learn and adapt with minimal human intervention, potentially reducing training time and costs. For example, DeepSeek-R1, a reasoning-focused model, was trained using pure reinforcement learning, allowing it to develop advanced reasoning capabilities with minimal human intervention.
DeepSeek also utilizes synthetic training data, as seen in the development of DeepSeek-R1, which was partially trained on "thinking" scripts generated by OpenAI's o1 model. This innovative use of synthetic data further reduces the reliance on expensive, human-created datasets. By using OpenAI's o1 model to generate these scripts, DeepSeek leverages existing AI capabilities to create its own training data, potentially lowering costs and accelerating development.
Another key aspect of DeepSeek's training efficiency is the use of Multi-Token Prediction (MTP) training. Instead of predicting one token at a time, DeepSeek V3 uses MTP, allowing the model to predict multiple tokens in parallel. This improves efficiency and potentially speeds up inferen
-iXYCNQmc9796KG6niP2xMACPwsE11U.png)
DeepSeek Model Portfolio
DeepSeek has developed a diverse portfolio of AI models, each with unique features and capabilities:
- DeepSeek Coder: Designed for coding tasks, trained on a mix of code and natural language data.
- DeepSeek LLM: A general-purpose large language model with broad language understanding capabilities.
- DeepSeek-V2: Introduced Multi-head Latent Attention and DeepSeekMoE architecture for efficient inference and training.
- DeepSeek-Coder-V2: Expanded coding capabilities with a larger context window and support for multiple programming languages.
- DeepSeek-V3: A milestone model with advanced mixture-of-experts architecture and FP8 mixed precision training.
- DeepSeek-R1: Focuses on advanced reasoning capabilities, trained using pure reinforcement learning.
Janus-Pro: A multimodal model excelling in both understanding and text-to-image generation.
This diverse portfolio caters to a wide range of applications, from coding and language understanding to image generation and reasoning.
DeepSeek Deployment
DeepSeek offers flexible deployment options through Amazon Web Services (AWS):
- Amazon Bedrock Marketplace: Provides access to the DeepSeek-R1 model through a managed endpoint.
- Amazon SageMaker JumpStart: Offers pre-built solutions and workflows for deploying DeepSeek-R1.
- Amazon Bedrock Custom Model Import: Allows users to import and deploy DeepSeek-R1-Distill models.
- Amazon EC2 Trn1 instances: Provides access to specialized hardware optimized for running DeepSeek-R1.
These options cater to different needs and expertise levels, allowing users to deploy DeepSeek models in various environments.
Cost-Effective Pricing Strategies
DeepSeek's pricing strategies are designed to be accessible and competitive. The company offers affordable subscription plans, with API access starting at $0.14 per million input tokens. This granular pricing model allows users to pay only for the resources they consume, making it a cost-effective option for businesses and individuals. DeepSeek's pricing model is significantly more affordable than its competitors, particularly for developers and researchers.
DeepSeek vs. Competitors: A Cost Comparison
Feature | DeepSeek | OpenAI (GPT-4o) | Anthropic (Claude 3.5 Sonnet) | Google (Gemini 1.5 Pro) |
---|---|---|---|---|
Model Size | 671B parameters | Not available | Not available | Not available |
Training Cost | $5.58 million | $78 million 10 | $100 million | $191 million |
Inference Cost (per million tokens) | $0.14 - $2.19 | $1.25 - $10.00 | $3 - $15 | $1.25 - $10.00 |
Deployment Cost | $1.6 billion | Not available | Not available | Not available |
Open Source | Yes | No | No | No |
Note: DeepSeek's reported training cost of $5.58 million has been disputed, with some analysts estimating the actual cost to be significantly higher. However, even with these higher estimates, DeepSeek's training costs remain considerably lower than those of its competitors.
Data Privacy and Security Considerations
DeepSeek's data collection practices are extensive, encompassing various types of user data:
- Personal Information: This includes date of birth, email addresses, phone numbers, and passwords.
- User Content: Any content provided to the model, such as text, audio, and uploaded files.
- Hardware Information: IP addresses, phone models, and language settings.
- User Activity: Keystroke patterns, browsing history, and cookies.
This data is stored on China-based servers and may be used for various purposes, including research, development, and potential sharing with advertisers. These practices raise important considerations for user privacy and data security, particularly for users outside of China.
Conclusion
DeepSeek's cost-effectiveness stems from a combination of innovative architecture, efficient training methods, competitive pricing strategies, and flexible deployment options. Its models deliver high performance at a fraction of the cost of its competitors, making it a disruptive force in the AI industry. While uncertainties remain regarding the true cost of DeepSeek's development, its impact on the market is undeniable.
DeepSeek's cost-effectiveness has the potential to democratize access to advanced AI solutions, making them more widely available to businesses and individuals. This could lead to wider adoption of AI across various industries, fostering innovation and potentially accelerating the development of new AI applications. However, DeepSeek's data collection practices raise important questions about user privacy and data security, which need to be carefully considered.
As AI adoption continues to grow, DeepSeek's focus on efficiency and accessibility could reshape the landscape of AI applications, making advanced AI solutions more widely available and potentially influencing the direction of future AI development
Subscribe to Our Newsletter
Get the latest AI insights delivered weekly
We respect your privacy. Unsubscribe at any time.